Luna’s Low Cost Autonomous Coating Condition Monitoring System
Ethan Thompson, Kevin Farinholt
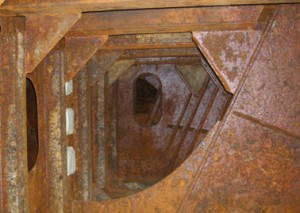
Approximately 4,000 ballast tanks across the U.S. Navy’s fleet must be inspected annually to determine their structural health and identify the presence of corrosion (Figure 1). These inspection efforts cost approximately $200 million dollars a year, with about 50% of the cost attributed to unexpected or unplanned repairs. The U.S. military is looking to transition from time-based inspection practices to condition-based maintenance for the next-generation Columbia-class nuclear submarines to reduce operating and maintenance costs.
Current maintenance practices involve visual inspections of the four major areas of the ballast tanks – tank tops, bottoms, sides, and T-beams, with four ballast tank locations common to most submarine designs (Figure 2). Ratings are given to each area as to the integrity of protective coatings and presence of corrosion, with ratings ranging from 0.03% - 10% deterioration. The overall condition of a tank is then assessed according to the rating for the most deteriorated region within the volume. Based on size and severity of damage, areas within the ballast tank will be flagged for mandatory repair or replacement should conditions exceed limits established within the Navy’s Corrosion Control Assessment and Maintenance Manual.
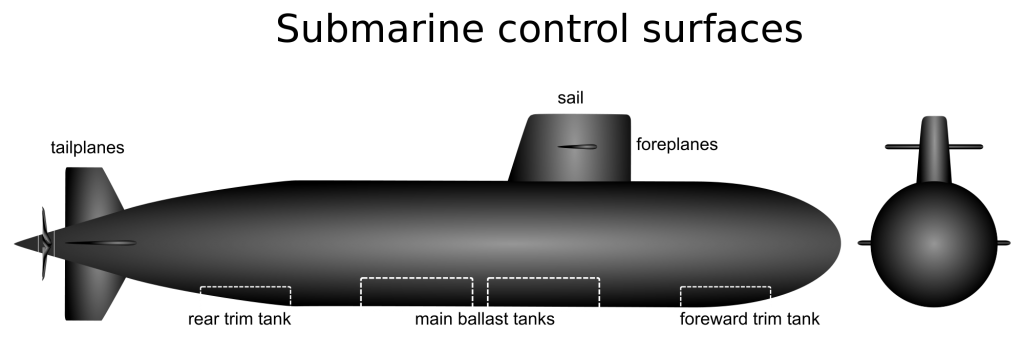
Luna is developing a technologically-rooted condition-based monitoring system for ballast tank coating damage (Figure 3). The development leverages hardware, software, and modeling techniques used across many of Luna’s corrosion and equipment health monitoring programs. The system incorporates autonomous monitoring of the interior protective coatings, and is expected to reduce labor cost, as well as provide operators a tool to understand the extent and location of the damage as it evolves. Luna has developed an embedded solution that utilizes a custom hardware design integrated with a graphical user interface to test and evaluate performance of the tank’s protective coating. Once data has been collected, it is fed through a neural network algorithm that estimates the defect size and position within the tank.
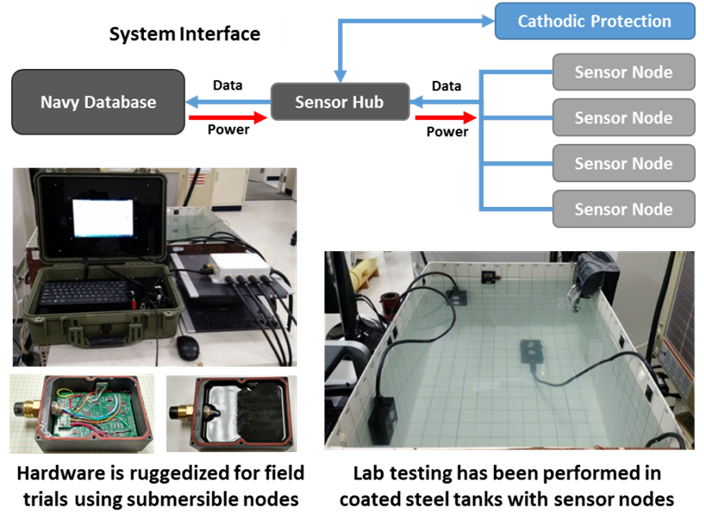
Electrochemical impedance spectroscopy (EIS) is used to monitor the electrical response of protective coatings and the saltwater contents they interact with within the ballast tank itself, collecting measurements over a frequency range from 500 Hz to 100 kHz. From these measurements, specific frequencies are correlated with defect size and separation distance for each of the sensor nodes. Data collected from multiple nodes is then transmitted to a central sensor hub where neural network algorithms are used to triangulate damage position and estimate its severity within the tank, providing an overall assessment for the tank (Figure 4). The system outputs a damage severity rating and provides maintainers locations within the tank that require further inspection or repair.
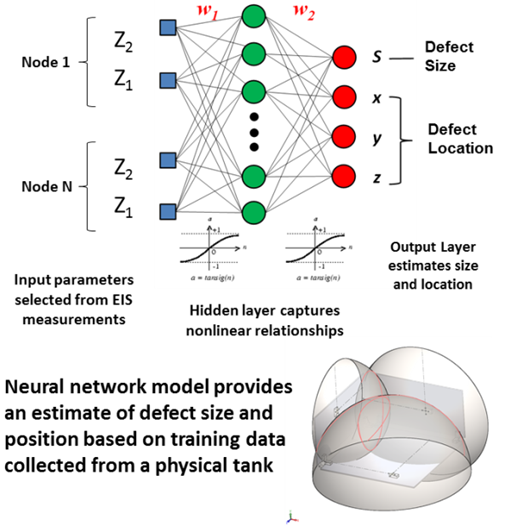
Experiments have been conducted on 1D, 2D, and 3D test stands in the laboratory. Physical scaled models of rectangular tanks have been fabricated for testing at Luna’s Charlottesville facility, and studied under a range of saltwater concentrations to simulate a range of operating conditions. Four- and five-node sensor arrays have been installed within each test stand, and a system was designed to quickly install and reposition simulated defects at different x-, y-, and z-coordinates along the walls, floor, and top of the tanks. As the sensor node collects data, a neural network model can be applied to generate estimates of the extent and location of damage within the tank (Figure 5). Training processes for the neural network model allow the system optimization to maximize prediction accuracy using both training and testing datasets collected in the laboratory. Results obtained to date have shown that the process can be applied to a variety of tests conditions with good performance at different salt concentrations, defect sizes, and spatial distributions.
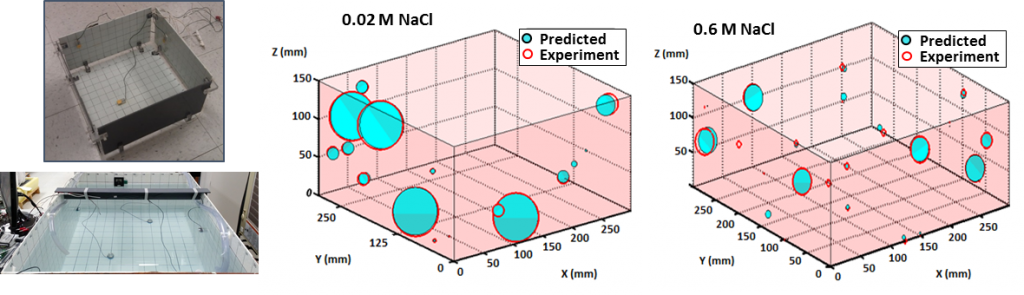
A ruggedized version of the coating condition monitoring system has been fabricated and is undergoing relevant environment testing at the U.S. Navy Research Laboratory’s Corrosion and Marine Engineering facility in Key West, Florida. Preliminary tests have been conducted to verify that EIS measurements are effective in larger expanses of natural seawater and separation distances up to 10m (Figure 6). These experiments were performed off of a seawall at NRL’s test facility where water is drawn from to fill ballast tank models constructed to study cathodic protection and monitoring systems.
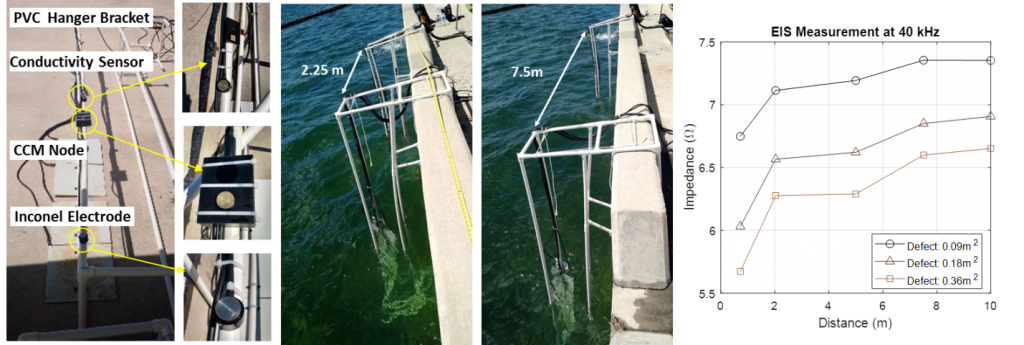
These field trials serve as a transition to relevant environment testing of Luna’s CCM system. This study will provide a realistic assessment of the technique’s ability to function in natural seawater environments, and at length scales that are representative of shipboard applications. They will also allow Luna to identify limitations of the current prototype system and implement system level refinements to mature the technology for further testing in operational environments to extend the technology readiness level of the coating condition monitoring system.